|
Mission Statement
Despite 40+ years of extensive research, software maintenance and evolution have never been easy. Studies show that software maintenance claims up to 60% of the total software budget.
Developers spend at least 50% of their time tackling various maintenance challenges (e.g., software bug resolution, new feature addition).
With the rise of popular but highly complex computational frameworks (e.g., Large Language Models, Deep learning, Cloud Computing, Mobile Computing), software maintenance has become even more difficult and costlier.
RAISE lab focuses on (a) a better understanding of software maintenance and evolution challenges (e.g., software bug resolution, new feature implementation) and
(b) designing intelligent, automated, and cost-effective software solutions to overcome these challenges and thus make the developers' lives easier. RAISE Lab has three signature areas as follows.
Signature Research Areas
Automated software debugging
Software bugs and failures are inevitable!
In 2017 alone, 606 software bugs cost the global economy $1.7 trillion+, with 3.7 billion people affected and 314 companies impacted!
While these bugs are ubiquitous, new classes of bugs are emerging due to the adoption of popular but highly complex technologies
such as Deep learning, Cloud computing, and Simulation models.
Many of these bugs are hidden not only in the software code but also in other artifacts such as configuration files, training data, trained models, deployment modules, and even simulation models.
Many of them are also non-deterministic and data-driven whereas the traditional software bugs are commonly logic-driven.
Thus, the traditional debugging techniques that have been developed for the last 40+ years might not be enough to tackle these emerging, complex bugs.
In the RAISE lab, we study the bugs from various complex software systems including machine learning software and simulation modelling software.
We attempt to (a) better understand the challenges of software debugging with a particular focus on bug/fault localization, bug understanding, and bug reproduction that take up ~90% of the debugging time, and
(b) design intelligent, explainable, cost-effective software solutions to overcome these challenges.
Automated code search
While finding and correcting bugs in the software is crucial, the majority of the maintenance budget (e.g., 60%) is spent incorporating new features in the existing software.
Once a software product is released, requests for new features from the users are very common. To stay competitive in the market,
the developers must add new, attractive features to their software at regular intervals.
However, modifying an existing software is not always straightforward.
A developer must know (a) how to implement a new feature and (b) where to add this feature within the software code.
Thus, as a part of feature addition, developers often search for reusable code snippets not only in the local codebase but also in the web.
Unfortunately, they often fail to make the right search queries.
According to existing surveys, they might even fail up to 73%-88% of the time, which is a significant waste of their valuable development time.
With the growing interest in prompt engineering in the context of large language models, search queries have been never more important.
In RAISE Lab, we develop state-of-the-art, intelligent tools and techniques that can
(a) automatically construct appropriate search queries and (b) find the desired code not only
from the local codebase but from the Internet-scale code repositories (e.g., GitHub, SourceForge).
Automated code review
Once software code is modified as a part of bug resolution or feature enhancement, the modified code must be checked for errors or quality issues.
Code review has been a vital quality-control practice for the last 30+ years where software code is manually checked by code reviewers (i.e., expert developers)
for hidden errors or quality issues. Finding the right reviewers and ensuring the high quality of code reviews are a challenging task.
Large software companies typically have large, geographically distributed development teams, which contain a tremendous pool of expertise
but are too big and diversified for manual assignment of review tasks. Incorrect selection of code reviewers not only delays the reviewing
process but also leads to poor reviews. Poorly written reviews often contain inaccurate suggestions and suffer from a lack of clarity or
empathy when pointing out mistakes in the software code, which makes the reviews ineffective. As a result, poor-quality reviews become a waste of
valuable development effort. Even at Microsoft Corporation, where 50,000+ professional developers spend six hours every week reviewing
each other’s code, ~35% of their code reviews are ineffective in improving software quality. Thus, modern code review needs appropriate
tools that can make it more productive and more efficient. In RAISE Lab, we design intelligent tools and techniques to (a) automatically find the right code reviewers, and
(b) automatically improve poor-quality code reviews.
Active Research Topics
- Bug Localization
- Bug Reproduction
- Bug Explanation
- Defect Prediction
- Duplicate Bug Detection
- Deep Learning Bugs
- Code Review Generation
- Patch Code Generation
- Search Query Reformulation
- Question-Answering
|
- Concept Location
- Internet-scale Code Search
- Crowdsourced Knowledge Mining
- Code Comprehension
- Recommendation Systems in Software Engineering
- IDE-based Meta Search Engine
- Exception Handling
- Software Re-documentation
|
Methodologies
- Generative AI and LLMs
- Information retrieval
- Machine/Deep learning
- Explainable AI
- Genetic Algorithms
- Mining software repositories
|
- Static code analysis
- Natural language processing
- Big Data analytics
- Grounded Theory
- Empirical studies
|
RAISE Team
|
Masud Rahman
Assistant Professor (Team Lead)
Faculty of Computer Science
Dalhousie University, Canada.
Research Interets: Please click here!
|
|
|
Sigma Jahan
PhD (Winter 2022 -- )
Research Interests: Bug localization, deep learning, transfer learning, and duplicate bug report detection.
|
|
Usmi Mukherjee
PhD (Winter 2024 -- )
Research Interests: Question-answering, information retrieval, generative AI models, and simulation models.
Co-supervised By: Dr. Ahsan Habib, Dalhousie University.
|
|
|
Asif Samir
PhD (Fall 2022 -- )
Research Interests: Bug localization, question-answering, generative AI models, and information retrieval.
|
|
Mehil Shah
PhD (Winter 2023 -- )
Research Interests: Bug reproduction, bug localization, machine learning, and deep learning.
Co-supervised By: Dr. Foutse Khomh, Polytechnique Montreal.
|
|
|
Riasat Mahbub
MCS (Fall 2023 -- )
Research Interests: Software debugging, simulation models.
Co-supervised By: Dr. Ahsan Habib, Dalhousie University.
|
|
Md Nizamuddin
BCS (Spring 2024 -- )
Research Interests: Software bug reports, natural language processing, and Domain term classification.
|
|
RAISE Graduates
|
Parvez Mahbub
MCS (Summer 2023)
Thesis: Comprehending Software Bugs Leveraging Code Structures with Neural Language Models.
Current Position: Software Engineer, Siemens Canada, Saskatoon
|
|
Ohiduzzaman Shuvo
MCS (Summer 2023)
Thesis: Improving Modern Code Review Leveraging Contextual and Structural Information from Source Code.
Current Position: Instructor, CIOT, Calgary
|
|
|
Usmi Mukherjee
MCS (Fall 2023)
Thesis: Complementing Deficient Bug Reports with Missing Information Leveraging Neural Text Generation.
Current Position: PhD Student, Dalhousie University.
|
|
Callum MacNeil
BCS (Fall 2023)
Thesis: A Systematic Review of Automated Program Repair using Large Language Models.
Current Position: MCS Student, Dalhousie University.
|
|
|
Shihui Gao
BCS (Fall 2023)
Thesis: Code Search in the IDE with Query Reformulation.
Current Position: Data Analyst, EMC, Halifax.
|
|
Lareina Yang
BCS (Winter 2023 -- )
Thesis: Search Term Identification for Concept Location Leveraging Word Relations.
Current Position: MS Student, Cornell University, New York.
|
|
Join RAISE!
RAISE Lab has been looking for a highly motivated, hard working individual for one PhD position. The candidate should have excellent academic background (e.g., CGPA: 3.7/4.00),
strong communication/writing skills, and an evidence of research excellence.
If you are enthusiastic about solving real-life Software Engineering problems and your credentials meet these requirements,
please send your (1) CV (including major achievements), (2) BSc/MSc transcripts, (3) IELTS/TOEFL scores (more details),
(4) sample publications, and (5) one-page summary of your past research excellence to
masud[DOT]rahman[AT]dal[DOT]ca .
The one-page summary should also include how your past experience might align with our research goals and interests.
It should be noted that only potentially eligible candidates might be contacted for an interview due to a high volume of applications.
More details about the admission into Dalhousie University.
Recent Projects
Explaining Software Bugs Leveraging Code Structures in Neural Machine Translation (ICSE 2023, ICSME 2023)
Overview:
Software bugs claim ≈ 50% of development time and cost the global economy billions of dollars. Over the last five decades, there has been significant research on automatically finding or correcting software bugs.
However, there has been little research on automatically explaining the bugs to the developers, which is a crucial but highly challenging task.
To fill this gap, we developed Bugsplainer, a transformer-based generative model, that generates natural language explanations for software bugs by learning from a large corpus of bug-fix commits.
Bugsplainer can leverage structural information and buggy patterns from the source code to generate an explanation for a bug. A developer study involving 20 participants shows that the explanations
from Bugsplainer are more accurate, more precise, more concise and more useful than the baselines. Explore more ...
bug-explanation deep-learning neural-text-generation transformer-based-model
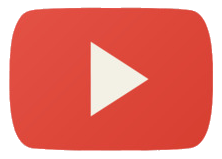
A Systematic Review of Automated Query Reformulations in Source Code Search (TOSEM 2023)
Overview: In this systematic literature review, we carefully select 70 primary studies on query reformulations from 2,970 candidate studies, perform an in-depth qualitative analysis using the Grounded Theory approach, and then answer seven important research questions. Our investigation has reported several major findings. First, to date, eight major methodologies (e.g., term weighting, query-term co-occurrence analysis, thesaurus lookup) have been adopted in query reformulation. Second, the existing studies suffer from several major limitations (e.g., lack of generalizability, vocabulary mismatch problem, weak evaluation, the extra burden on the developers) that might prevent their wide adoption.
Finally, we discuss several open issues in search query reformulations and suggest multiple future research opportunities. Explore more ...
query-reformulation bug-localization concept-location code-search empirical-study
grounded-theory
Towards Understanding the Impacts of Textual Dissimilarity on Duplicate Bug Report Detection (SANER 2023)
Overview: A large-scale empirical study using 92K bug reports from three open-source systems is done to understand the challenges of textual dissimilarity in duplicate bug report detection. First, empirical evidence is demonstrated using existing techniques that poorly detect textually dissimilar duplicate bug reports. Second, we found that textually dissimilar duplicates often miss essential components (e.g., steps to reproduce), which could lead to their textual dissimilarity within the same pair.
Finally, inspired by the earlier findings, domain-specific embedding along with CNN is applied to duplicate bug report detection, which provides mixed results. Explore more ...
information-retrieval deep-learning duplicate-bug-detection empirical-study
Recommending Code Reviews Leveraging Code Changes with Structured Information Retrieval (ICSME 2023)
Overview: Review comments are one of the main building blocks of modern code reviews. Manually writing code review comments could be time-consuming and technically challenging. In this work, we propose a novel technique for relevant review comments recommendation -- RevCom -- that leverages various code-level changes using structured information retrieval. It uses different structured items from source code and can recommend relevant reviews for all types of changes (e.g., method-level and non-method-level). We find that RevCom can recommend review comments with an average BLEU score of ≈ 26.63%. According to Google's AutoML Translation documentation, such a BLEU score indicates that the review comments
can capture the original intent of the reviewers. Our approach is lightweight compared to DL-based techniques and can recommend reviews for both method-level and non-method-level changes where the existing IR-based technique falls short. Explore more ...
structured-information-retrieval code-review-automation
The Forgotten Role of Search Queries in IR-based Bug Localization: An Empirical Study [EMSE 2021, ICSE 2022 (Journal First)]
Overview: We conduct an in-depth empirical study that critically examines the state-of-the-art query selection practices in IR-based bug localization. In particular, we use a dataset of 2,320 bug reports, employ ten existing approaches from the literature, exploit the Genetic Algorithm-based approach to construct optimal, near-optimal search queries from these bug reports, and then answer three research questions. We confirmed that the state-of-the-art query construction approaches are indeed not sufficient for constructing appropriate queries (for bug localization) from certain natural language-only bug reports although they contain such queries. We also demonstrate that optimal queries and non-optimal queries chosen from bug report texts are significantly different in terms of several keyword characteristics, which has led us to actionable insights. Furthermore, we demonstrate 27%--34% improvement in
the performance of non-optimal queries through the application of our actionable insights to them. Explore more ...
query-reformulation bug-localization empirical-study
genetic-algorithm
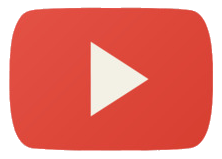
Why Are Some Bugs Non-Reproducible? An Empirical Investigation using Data Fusion [ICSME 2020, EMSE 2022]
Overview: We conduct a multimodal study to better understand the non-reproducibility of software bugs.
First, we perform an empirical study using 576 non-reproducible bug reports from two popular software systems (Firefox, Eclipse) and identify 11 key factors that might lead a reported bug to non-reproducibility. Second, we conduct a user study involving 13 professional developers where we investigate how the developers cope with non-reproducible bugs. We found that they either close these bugs or solicit for further information, which involves long deliberations and counter-productive manual searches. Third, we offer several actionable insights on how to avoid non-reproducibility (e.g., false-positive bug report detector) and improve reproducibility of the reported bugs (e.g., sandbox for bug reproduction) by combining our analyses from multiple studies (e.g., empirical study, developer study).
Explore more ...
empirical-study data-fusion bug-reproduction
grounded-theory
Automated Software Debugging
Explaining Software Bugs Leveraging Code Structures in Neural Machine Translation (ICSE 2023)
Overview:
Software bugs claim ≈ 50% of development time and cost the global economy billions of dollars. Over the last five decades, there has been significant research on automatically finding or correcting software bugs.
However, there has been little research on automatically explaining the bugs to the developers, which is a crucial but highly challenging task.
To fill this gap, we developed Bugsplainer, a transformer-based generative model, that generates natural language explanations for software bugs by learning from a large corpus of bug-fix commits.
Bugsplainer can leverage structural information and buggy patterns from the source code to generate an explanation for a bug. A developer study involving 20 participants shows that the explanations
from Bugsplainer are more accurate, more precise, more concise and more useful than the baselines.
bug-explanation deep-learning neural-text-generation transformer-based-model
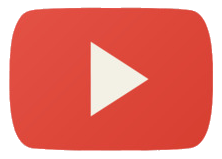
A Systematic Review of Automated Query Reformulations in Source Code Search (TOSEM 2023)
Overview: In this systematic literature review, we carefully select 70 primary studies on query reformulations from 2,970 candidate studies, perform an in-depth qualitative analysis using the Grounded Theory approach, and then answer seven important research questions. Our investigation has reported several major findings. First, to date, eight major methodologies (e.g., term weighting, query-term co-occurrence analysis, thesaurus lookup) have been adopted in query reformulation. Second, the existing studies suffer from several major limitations (e.g., lack of generalizability, vocabulary mismatch problem, weak evaluation, the extra burden on the developers) that might prevent their wide adoption.
Finally, we discuss several open issues in search query reformulations and suggest multiple future research opportunities. Explore more ...
query-reformulation bug-localization concept-location code-search empirical-study
grounded-theory
Towards Understanding the Impacts of Textual Dissimilarity on Duplicate Bug Report Detection (SANER 2023)
Overview: A large-scale empirical study using 92K bug reports from three open-source systems is done to understand the challenges of textual dissimilarity in duplicate bug report detection. First, empirical evidence is demonstrated using existing techniques that poorly detect textually dissimilar duplicate bug reports. Second, we found that textually dissimilar duplicates often miss essential components (e.g., steps to reproduce), which could lead to their textual dissimilarity within the same pair.
Finally, inspired by the earlier findings, domain-specific embedding along with CNN is applied to duplicate bug report detection, which provides mixed results. Explore more ...
information-retrieval deep-learning duplicate-bug-detection empirical-study
The Forgotten Role of Search Queries in IR-based Bug Localization: An Empirical Study [EMSE 2021, ICSE 2022 (Journal First)]
Overview: We conduct an in-depth empirical study that critically examines the state-of-the-art query selection practices in IR-based bug localization. In particular, we use a dataset of 2,320 bug reports, employ ten existing approaches from the literature, exploit the Genetic Algorithm-based approach to construct optimal, near-optimal search queries from these bug reports, and then answer three research questions. We confirmed that the state-of-the-art query construction approaches are indeed not sufficient for constructing appropriate queries (for bug localization) from certain natural language-only bug reports although they contain such queries. We also demonstrate that optimal queries and non-optimal queries chosen from bug report texts are significantly different in terms of several keyword characteristics, which has led us to actionable insights. Furthermore, we demonstrate 27%--34% improvement in
the performance of non-optimal queries through the application of our actionable insights to them. Explore more ...
query-reformulation bug-localization empirical-study
genetic-algorithm
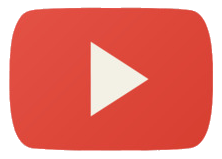
Why Are Some Bugs Non-Reproducible? An Empirical Investigation using Data Fusion [ICSME 2020 + EMSE 2022]
Overview: We conduct a multimodal study to better understand the non-reproducibility of software bugs.
First, we perform an empirical study using 576 non-reproducible bug reports from two popular software systems (Firefox, Eclipse) and identify 11 key factors that might lead a reported bug to non-reproducibility. Second, we conduct a user study involving 13 professional developers where we investigate how the developers cope with non-reproducible bugs. We found that they either close these bugs or solicit for further information, which involves long deliberations and counter-productive manual searches. Third, we offer several actionable insights on how to avoid non-reproducibility (e.g., false-positive bug report detector) and improve reproducibility of the reported bugs (e.g., sandbox for bug reproduction) by combining our analyses from multiple studies (e.g., empirical study, developer study).
Explore more ...
empirical-study data-fusion bug-reproduction
grounded-theory
BugDoctor: Intelligent Search Engine for Software Bugs and Features [ICSE-C 2019]
Overview: Bug Doctor assists the developers in localizing the software code of interest (e.g., bugs, concepts and reusable code) during software maintenance.
In particular, it reformulates a given search query (1) by designing a novel keyword selection algorithm (e.g., CodeRank)
that outperforms the traditional alternatives (e.g., TF-IDF),
(2) by leveraging the bug report quality paradigm and source document structures which were previously overlooked and
(3) by exploiting the crowd knowledge and word semantics derived from Stack Overflow Q&A site, which were previously untapped.
An experiment using 5000+ search queries (bug reports, change requests, and ad hoc queries) suggests
that Bug Doctor can improve the given queries significantly through automated query reformulations.
Comparison with 10+ existing studies on bug localization, concept location and Internet-scale code
search suggests that Bug Doctor can outperform the state-of-the-art approaches with a significant margin.
Explore more ...
query-reformulation bug-localization concept-location code-search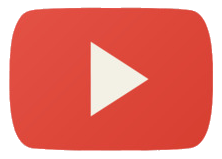
|
BLIZZARD: Improving IR-Based Bug Localization with Context-Aware Query Reformulation [ESEC/FSE 2018]
Overview: BLIZZARD is a novel technique for IR-based bug localization that uses query reformulation and bug report quality dynamics.
We first conduct an empirical study to analyse the report quality dynamics of bug reports and then design an IR-based bug localization technique using
graph-based keyword selection, query reformulation, noise filtration, and Information Retrieval. Explore more ...
query-reformulation bug-localization
CodeInsight: Recommending Insightful Comments for Source Code using Crowdsourced Knowledge [SCAM 2015]
Overview: CodeInsight is an automated technique for generating insightful comments for source code using crowdsourced knowledge from Stack Overflow.
It uses data mining, topic modelling, sentiment analysis and heuristics for deriving the code-level insights.
Explore more ...
data-mining stack-overflow
Automated Code Review
Automated Code Search
STRICT: Search Term Identification for Concept Location using Graph-Based Term Weighting [SANER 2017]
Overview: STRICT is a novel technique for identifying appropriate search terms from a software change request.
It uses graph-based term weighting (PageRank), natural language processing and Information Retrieval to identify the important keywords and
then finds the code of interest (e.g., software feature). Explore more ...
query-reformulation concept-location
ACER: Improved Query Reformulation for Concept Location using CodeRank and Document Structures [ASE 2017]
Overview: ACER offers effective reformulations to queries for concept location using CodeRank and source document structures.
It uses graph-based keyword selection from source code, query difficulty analysis, machine learning and Information Retrieval for reformulating the queries.
Explore more ...
query-reformulation concept-location
RACK: Automatic Query Reformulation for Code Search using Crowdsourced Knowledge [SANER 2016 + EMSE 2019 + ICSE 2017]
|
Overview: We propose a novel query reformulation technique--RACK--that suggests a list of relevant API classes for a natural language query intended for code search. Our technique offers such suggestions by exploiting keyword-API associations from the questions and answers of Stack Overflow (i.e., crowdsourced knowledge).
We first motivate our idea using an exploratory study with 19 standard Java API packages and 344K Java related posts from Stack Overflow. Experiments using 175 code search queries randomly chosen from three Java tutorial sites show that our technique recommends correct API classes within the Top-10 results for 83% of the queries, with 46% mean average precision and 54% recall, which are 66%, 79% and 87% higher respectively than that of the state-of-the-art. Reformulations using our suggested API classes improve 64% of the natural language queries and their overall accuracy improves by 19%. Comparisons with three state-of-the-art techniques demonstrate that RACK outperforms them in the query reformulation by a statistically significant margin. Investigation using three web/code search engines shows that our technique can significantly improve their results in the context of code search.
Explore more ...
query-reformulation code-search stack-overflow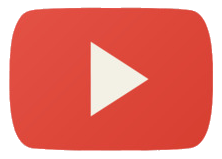
|
NLP2API: Effective Reformulation of Query for Code Search using Crowdsourced Knowledge and Extra-Large Data Analytics [ICSME 2018]
Overview: NLP2API reformulates a natural language query intended for Internet-scale code search using
crowdsourced knowledge and extra-large data analytics derived from Stack Overflow Q & A site. Explore more ...
query-reformulation code-search stack-overflow
BLADER: Improving Queries for IR-Based Bug Localization with Semantics-Driven Query Reformulation [Ongoing Work]
Overview: BLADER complements a bug report that does not
contain any structured entities (e.g., program entity names) and uses the complemented report as a query for IR-based bug localization.
It employs word embeddings derived from Stack Overflow with FastText for the query reformulation. Explore more ...
query-reformulation bug-localization
SurfClipse: Context-Aware IDE-Based Meta Search Engine for Programming Errors & Exceptions [CSMR-WCRE 2014 + ICSME 2014 + WCRE 2013]
|
Overview:
We propose a context-aware meta search tool, SurfClipse, that analyzes an encountered exception andits context in the IDE, and recommends not only suitable search queries but also relevant web pages for the exception (and its context). The tool collects results from three popular search engines and a programming Q & A site against the exception in the IDE, refines the results for relevance against the context of the exception, and then ranks them before recommendation. It provides two working modes--interactive and proactive to meet the versatile needs of the developers, and one can browse the result pages using a
customized embedded browser provided by the tool. Explore more ...
recommendation-system search-engine stack-overflow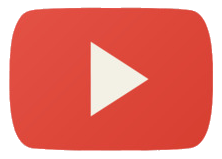
|
ExcClipse: Context-Aware Meta Search Engine for Programming Errors and Exceptions
|
Overview: In this MSc thesis, we develop a context-aware, IDE-based, meta search engine --ExcClipse-- that delivers relevant web pages and code examples within the IDE panel
for dealing with programming errors and exceptions. Once a programming error/exception is encountered, the tool (1) constructs an appropriate query by capturing
the error details and meta data, (2) collects results from popular search engines--Google, Bing, Yahoo, StackOverflow and GitHub,
(3) refines and ranks the results against the context of the encountered exception, and (4) then recommends them within the IDE.
We develop our solution as an Eclipse plug-in prototype. Explore more ...
recommendation-system search-engine stack-overflow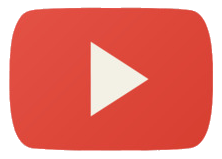
|
Automated Q&A
Under construction. Please come back later.
Theses & Dissertations
Parvez Mahbub (MCS). Comprehending Software Bugs Leveraging Code Structures with Neural Language Models (Summer 2023)
Overview: This thesis introduces Bugsplorer, a deep-learning technique for line-level defect prediction, demonstrating 26-72% better accuracy than existing methods and efficient ranking of vulnerable lines.
Additionally, Bugsplainer, a transformer-based generative model, provides natural language explanations for software bugs, outperforming multiple baselines according to evaluation metrics and a developer study with 20 participants.
The empirical evidence suggests that these techniques have the potential to substantially reduce Software Quality Assurance costs. Explore more ...
bug-explanation deep-learning neural-text-generation transformer-based-model
Ohiduzzaman Shuvo (MCS). Improving Modern Code Review Leveraging Contextual and Structural Information from Source Code (Summer 2023)
Overview: This thesis first conducts an empirical study, revealing significant performance variations in existing techniques for assessing code reviews between open-source and closed-source systems.
The study indicates that less experienced developers tend to submit more non-useful review comments in both contexts, emphasizing the need for automated support in code review composition.
To help developers write better review comments, another technique namely RevCom was proposed. RevCom utilizes structured information retrieval and outperforms both Information Retrieval (IR)-based and Deep Learning (DL)-based baselines,
offering a lightweight and scalable solution with the potential to alleviate cognitive effort and save time for reviewers. Explore more ...
structured-information-retrieval code-review-automation
Usmi Mukherjee (MCS). Complementing Deficient Bug Reports with Missing Information Leveraging Neural Text Generation (Fall 2023)
Overview: This thesis introduces and assesses two novel Generative AI approaches for enhancing deficient bug reports. The first approach, BugMentor, utilizes structured information retrieval and neural text generation
to provide contextually appropriate answers to follow-up questions in bug reports, demonstrating superior performance over three existing baselines in terms of metrics such as BLEU and Semantic Similarity.
A developer study further validates BugMentor's effectiveness in generating more accurate, precise, concise, and useful answers. The second approach, BugEnricher, fine-tunes a T5 model on software-specific vocabulary to generate
meaningful explanations, outperforming two baselines and showing promise in improving the detection of textually dissimilar duplicate bug reports, a known challenge in bug report management.
The empirical evidence suggests these approaches hold strong potential for supporting bug resolution and enhancing bug report management. Explore more ...
bug-report-enhancement deep-learning neural-text-generation transformer-based-model
Lareina Yang (BCS). Search Term Identification for Concept Location Leveraging Word Relations (Winter 2024)
Overview: The thesis extends an existing approach called STRICT to improve keyword selection for software change requests using graph-based algorithms like TextRank, POSRank, SimRank, Biased TextRank, and PositionRank. Experiments show that
the enhanced approach, STRICT++, outperforms STRICT in detecting software bugs, with significant performance improvements in metrics like MAR, MRR, and Top-10 Accuracy. The thesis emphasizes the importance of
capturing syntactic relationships and considers factors like word similarity,
task-specific biases, and position information for effective keyword extraction. Explore more ...
bug-localization concept-location query-reformulation information-retrieval
Callum MacNeil (BCS). A Systematic Review of Automated Program Repair using Large Language Models (Fall 2023)
Overview: This thesis conducts a systematic review of automated program repair tools leveraging large language models, scrutinizing 1,276 papers published between 2017 and 2023 and
narrowing down the analysis to 53 primary studies. The findings indicate a prevalent choice among these studies to utilize popular datasets and pre-trained models, specifically Defects4j and codeT5.
The review reveals a tendency for studies to target specific aspects of program repair, such as validation or input representation, and underscores challenges in input representation, evaluation methods,
and learning objectives, emphasizing the pivotal trade-off between efficacy and efficiency. Leveraging this comprehensive understanding, the thesis identifies future directions and best approaches
for research on automated program repair using large language models. Explore more ...
bug-fixing deep-learning neural-code-generation transformer-based-model
Disclaimer: The overview of each thesis has been generated from their original abstracts using ChatGPT.
Outreach, Milestones, & Social
|
Collaborators & Partners
|